GPTQ LLM Leaderboard Report #5
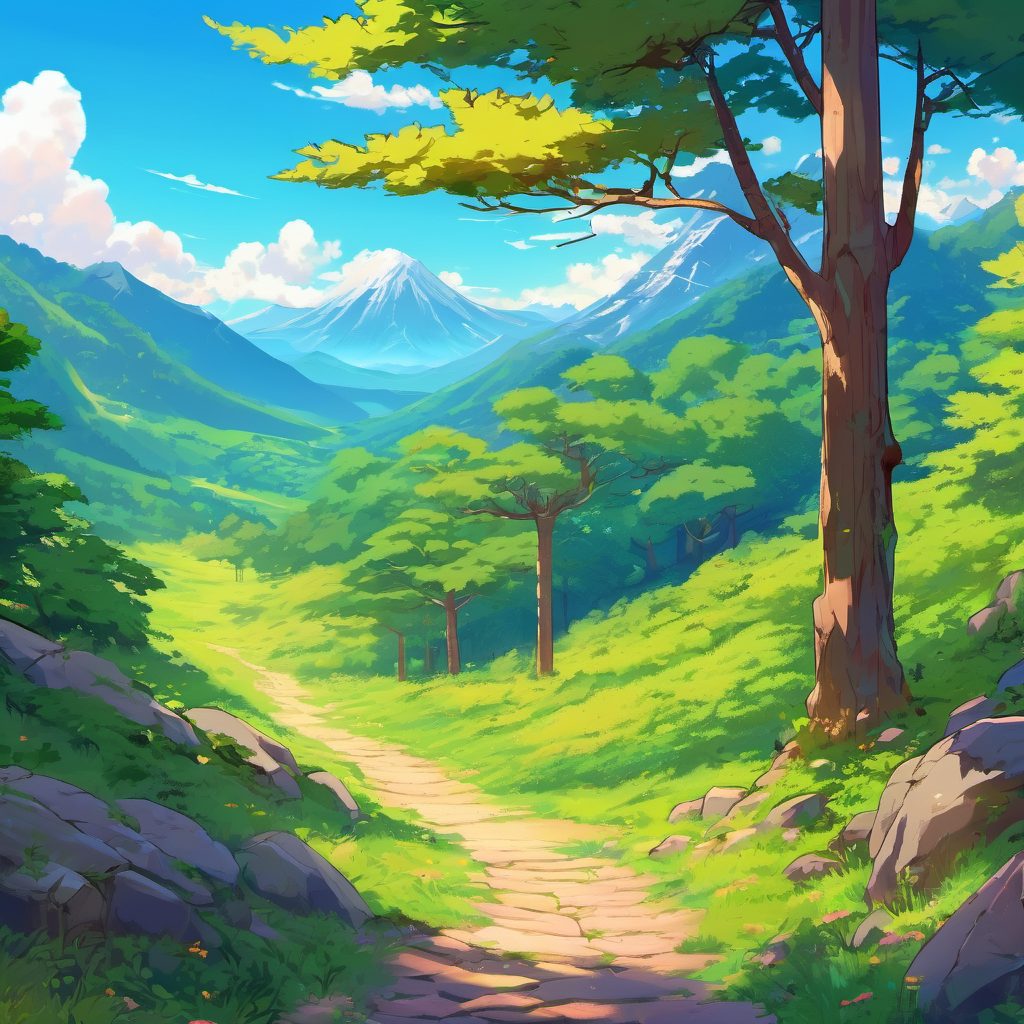
I've been playing with SDXL LoRA and Fine-tuning these past few weeks and I'm telling you, it's very hard to get right. LoRA seems easier but there are a lot of tweaks that can make it better. In the mean time, I've finished evaluating a handful of GPTQ models including the new OpenOrca x OpenChat model. Following are the results.
Models Tested
- TheBloke/openchat_v2_openorca_preview-GPTQ (main)
- TheBloke/OpenOrcaxOpenChat-Preview2-13B-GPTQ (main)
- TheBloke/OpenOrcaxOpenChat-Preview2-13B-GPTQ (gptq-4bit-32g-actorder_True)
- TheBloke/OpenOrcaxOpenChat-Preview2-13B-GPTQ (gptq-8bit-128g-actorder_True)
- TheBloke/StableBeluga-13B-GPTQ (main)
- TheBloke/StableBeluga-13B-GPTQ (gptq-4bit-32g-actorder_True)
- TheBloke/StableBeluga-13B-GPTQ (gptq-8bit-128g-actorder_True)
- TheBloke/Vigogne-2-13B-Instruct-GPTQ (main)
- TheBloke/Vigogne-2-13B-Instruct-GPTQ (gptq-4bit-32g-actorder_True)
- TheBloke/Vigogne-2-13B-Instruct-GPTQ (gptq-8bit-64g-actorder_True)
Results
You can see all the results here.
The most VRAM hungry model of this report is TheBloke/Vigogne-2-13B-Instruct-GPTQ (gptq-8bit-64g-actorder_True), uses up to 16965 MBs of VRAM.
The least VRAM hungry model of this report is TheBloke/openchat_v2_openorca_preview-GPTQ (main), which uses up to 8.4 GBs of VRAM.